Explainable AI
What is Explainable AI?
Explainable artificial intelligence or explainable AI (sometimes known as the shorthand “XAI”) refers to the ability of algorithm or model owners to understand how AI reached its findings by making AI technology as transparent as possible. With explainable AI – as well as interpretable machine learning – organizations gain access to AI technology’s underlying decision-making and are empowered to make adjustments as needed.
Why Is Explainable AI Important?
A common concern among potential artificial intelligence adopters is that it is often unclear how the technology reaches its conclusions. When AI algorithms are locked in a “black box” preventing humans from analyzing how the findings were reached, it can be difficult to trust the technology because human experts are unable to explain the AI’s findings.
Being able to explain AI can help organizations establish greater trust with clients, customers, and invested stakeholders. One of the key benefits of being able to have artificial intelligence explained simply is that it can help technology owners determine if human bias influenced the model. This can be especially important when the AI is being called upon to make life-or-death decisions, such as in a hospital environment where medical professionals may have to explain to patients and their families why certain decisions were made.
Take the example of a healthcare system that was designed to determine whether a patient will need additional medical resources by assigning a “commercial risk score” to determine the level of care management a patient should receive. When medical professionals gained access to proprietary data, they discovered that the algorithm was more likely to measure healthcare costs rather than illness. As a result, researchers learned that zip codes were a leading predictor of patients’ hospital stays. The zip codes that correlated to longer hospital stays tended to be in poor and predominantly African-American neighborhoods. When mapped by commercial risk scores versus the number of active chronic conditions split by race, researchers discovered that African-American patients with the same number of chronic health problems received lower commercial risk scores and, as a result, less care.
In other words, explainable AI helped healthcare providers pinpoint how human bias built directly into their AI was impacting patient care. In addition to the healthcare market, this level of transparency can allow individuals impacted by the European Union’s Global Data Protection Regulation (GDPR) or the U.K.’s Data Protection Bill to exercise the “right to explanation” as to how data was used by an algorithm. These are just a few examples of how explainable AI can make regulated markets – banking, healthcare, and insurance, to name a few – more transparent and trustworthy.
Explainable AI + DataRobot
DataRobot offers a model-agnostic framework that enables owners to interpret results, make informed adjustments, and access easy-to-use and state-of-the-art interpretation techniques for all their models. This promotes consistent techniques across all models instead of different approaches for different models that can result in biased decision-making. Having this level of transparency empowers firms to meet the “right to explanations” for end-users, provide stakeholders with explanations into a model’s logic, and improve compliance with existing regulations.
DataRobot’s team of consumer facing data scientists can not only help your organization become AI-driven, but driven by explainable AI. Meanwhile, our R&D team is constantly growing and testing its library of AI and machine learning models and provides documents outlining each step a model uses to reach its conclusions to help you trust your AI and explain it to your stakeholders. Here are some key features that DataRobot can offer:
- Feature Impact: Shows how much a model relies on each individual feature to reach its decisions.
- Feature Effects: Enables users to delve deeper into models and investigate how feature values influence the model’s decision on a global level.
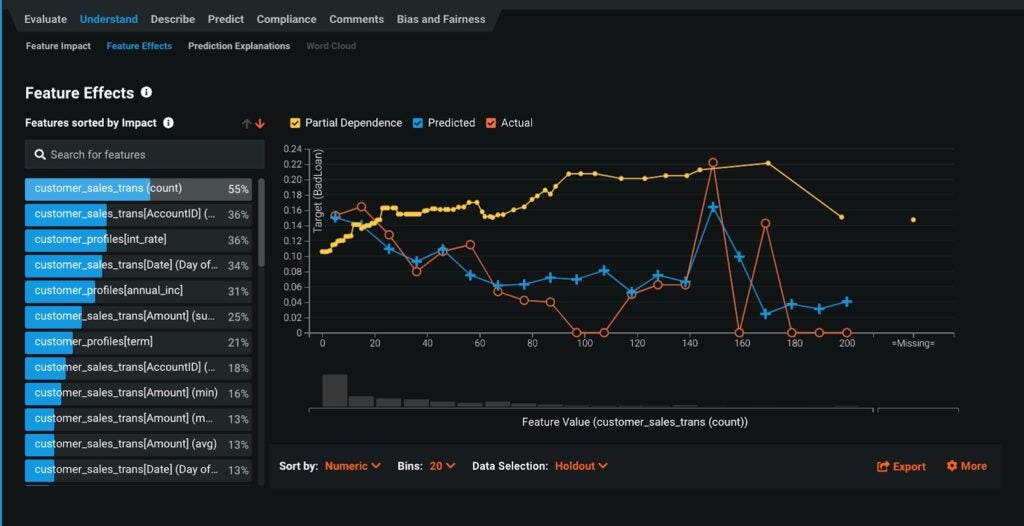
- Prediction Explanation: Highlights the features variables that impact each model’s decision outcome for each record and the magnitude of different features for each.
DataRobot automates several standard data processing steps within each model blueprint and makes all these transformations transparent. This ensures that AI models are not locked in a black box, a common problem that can arise when organizations turn to third-party technology suppliers to address their AI solutions. Our products are designed to help your organization build trustworthy AI models for a wide array of use cases and to promote the democratization of data science and machine learning tools.