“We need to reinvent the way we market to customers. We need a new model.” A.G. Lafley, CEO, Proctor and Gamble, 2004
Have you ever received the same advertising, for the same products, over multiple communication sources?
Or maybe the same company sends you multiple offers, for different products, within only a few days of each other?
Have you ever been flooded with information or products which are not of interest to you?
That is often because companies have a wide range of product offerings and a large pool of customers. So, what are the traditional methods of getting the products out to market?
Method 1: Target all customers with all products.
This does not work because it might be seen as annoying and push customers away. Research shows that providing more choices might lower a customer’s satisfaction. (Harvard Business Review, June 2006)
Method 2: Group your customers into several categories and do targeted marketing in one of those categories.
This may work, but every customer is different, leading some to conclude that “non-demographic segmentation has become just as unenlightening as demographic segmentation” (Harvard Business Review, February 2006). Some customers might still be annoyed by this. Wouldn’t it be great if companies know exactly what you, as an individual, need or are interested in?
Solution: Tailor a Next Best Offer for each customer.
A next best offer is not new to marketers. However, it has recently gained traction due to various reasons, including:
- New regulations against spam.
- Customers being more tech-savvy and preferring to pull for product or service information themselves, rather than having it pushed at them.
Therefore, there is an increasing need to personalize the product or service for individual customers based on their needs.
The next best offer is also seen as the best practice by marketers. Some of the advantages include:
- Optimizing the marketing campaign value by having a targeted marketing campaign.
- Moving away from product-centric to customer-centric campaigns.
- Increasing customer satisfaction and brand value by avoiding spamming the customers.
The next best offer can be applied across many industries — ranging from products to services. In this post, we will show you how next best offer can be applied in a telecommunication company for cross-selling relevant mobile plans to their customers. In this example, the company can offer one of the four plans available, or they can choose to not take any action since there will be customers for whom none of these offers will be attractive, or who do not wish to be contacted.
We will be using machine learning to help us identify the next best offer. As the technique suggests, the machine will learn by example and find the best way to address our challenge. In order to do so, we will need to provide the machine with historical marketing campaign data, such as the plan offered to the customer and other factors (like usage patterns, demographics, or communication between the customer and the company).
Cust. ID |
Acct. Length |
Int. Plan |
Num. Vmail Messages |
Total Day Min. |
Total Eve. Min. |
Total Night Min. |
Total Intl. Min. |
Cust. Service Calls |
Offers |
1 |
128 |
No
|
25 |
265.1 |
197.4 |
244.7 |
10 |
1 |
No Action |
2 |
107 |
No |
26 |
161.6 |
195.5 |
254.4 |
13.7 |
1 |
Enhanced Voicemail Plan |
3 |
118 |
Yes |
0 |
223.4 |
220.6 |
203.9 |
6.3 |
0 |
Evening Call Plan |
4 |
121 |
No |
24 |
218..2 |
384.5 |
212.6 |
7.5 |
3 |
Evening Call Plan |
5 |
117 |
No |
0 |
184.5 |
351.6 |
215.8 |
8.7 |
1 |
Daytime Plan |
With this data, the machine will learn the characteristics of each individual account and build a machine learning model to predict the most appropriate offer for each customer. Thereafter, the company is able to apply the model to both new and existing customers, so they can cross-sell or upsell the most suitable product to their customers.
Cust. ID |
To Be Offered |
Probability |
3330 |
Daytime Plan |
0.97 |
3331 |
Intl. Call Plan |
0.83 |
3332 |
No Action |
0.67 |
3333 |
Intl. Call Plan |
0.47 |
However, knowing the prediction outcome may not be sufficient. With rising consumer activism, and GDPR legislation in the EU that require companies to provide explanations for algorithmic decisions, we will need to understand how the model works, and whether or not we can trust it.
The first thing to understand is what the model considers to be the most important factors. Do they make sense? We would not want a model that does not make any sense. For this example, what do you think of the features identified by the model? It appears that usage pattern is more important than where they live. From this analysis, we can gather initial insights on how each feature affects the prediction.
Figure 3: Feature Impact
The next question we would typically ask the model is, “How accurate is the prediction?” No prediction can achieve 100% accuracy. And in this use case, some customers prefer not to be contacted, even though there might be plans which could be beneficial to them. Each time you contact customers with the wrong offer, or when they are not interested in any offer, you damage your brand value. This would have a negative impact on the retention rate, thus reducing the lifetime value of the customer.
With the next best offer model in place, marketers can focus on creating customized product or service offerings based on the individual profiles of each customer. Commonly, the product with the highest prediction score will be offered to the customer. Therefore, each customer will only receive one offer that best fits their profile — increasing the customer’s satisfaction and the brand value.
Does it sound too complicated to develop the next best offer model? Fret not! There are various tools in the market which can help. Some may need a data scientist to develop, but there is tool (that’s us, DataRobot) which empowers business analysts to perform the data scientist role, and frees up data scientists’ time from performing regimental work, allowing them to focus on value-added tasks.
So, let’s move to the next best offer world and treat each customer as an individual.
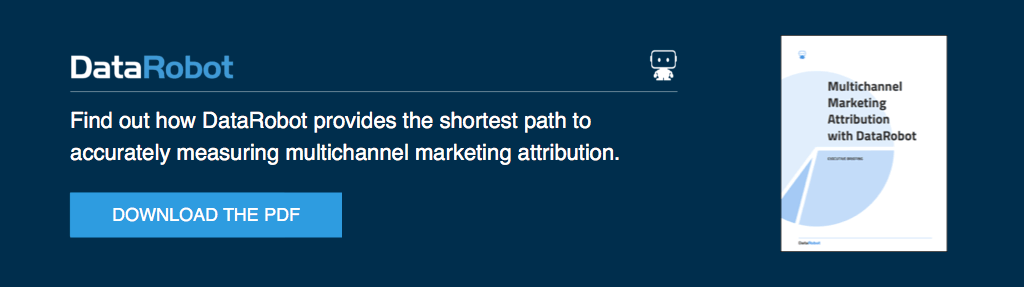
About the Author:
Yeo Hwee Theng is a Customer Facing Data Scientist at DataRobot, where she advises businesses on how to build business cases and address them from a data science perspective. Prior to DataRobot, Hwee Theng worked with multiple organisations across various industries such as financial services, retail services, and the public sector. With Hwee Theng’s expertise, organisations are able to turn data into actionable insights.
About the author
Hwee Theng Yeo
Customer Facing Data Scientist, DataRobot
Hwee Theng Yeo is a Customer-Facing Data Scientist (CFDS) at DataRobot working in Singapore.
Meet Hwee Theng Yeo