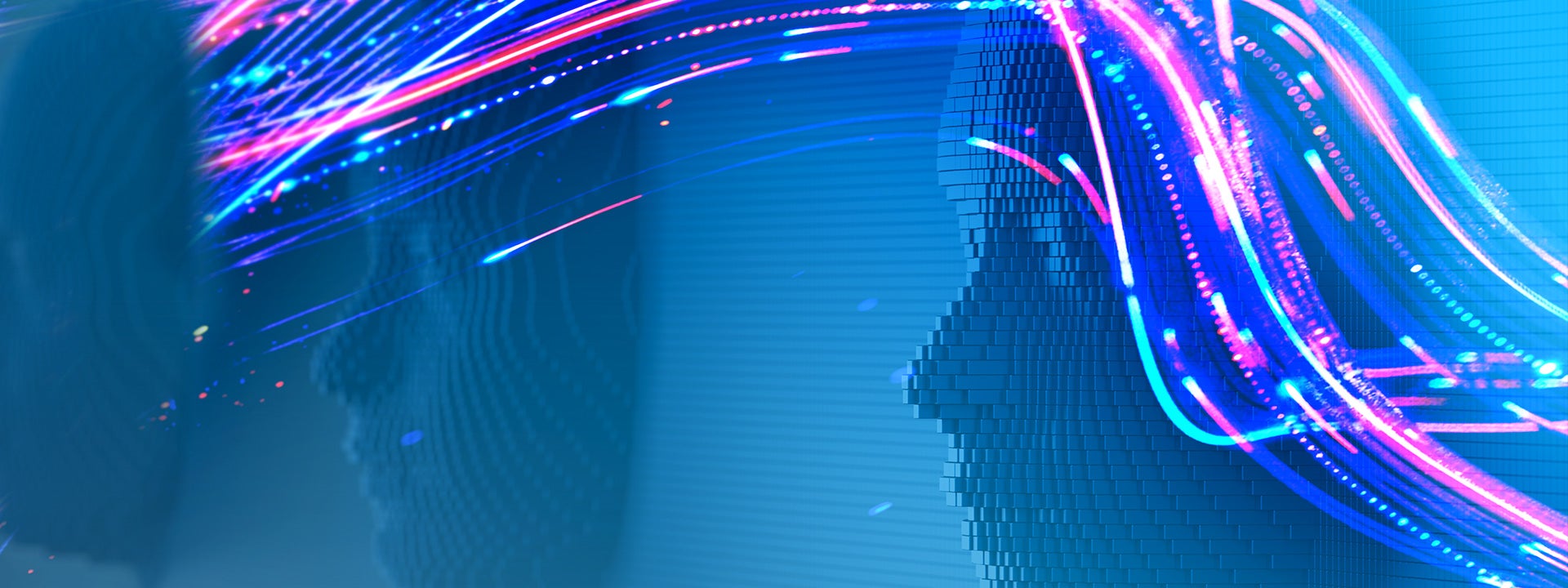
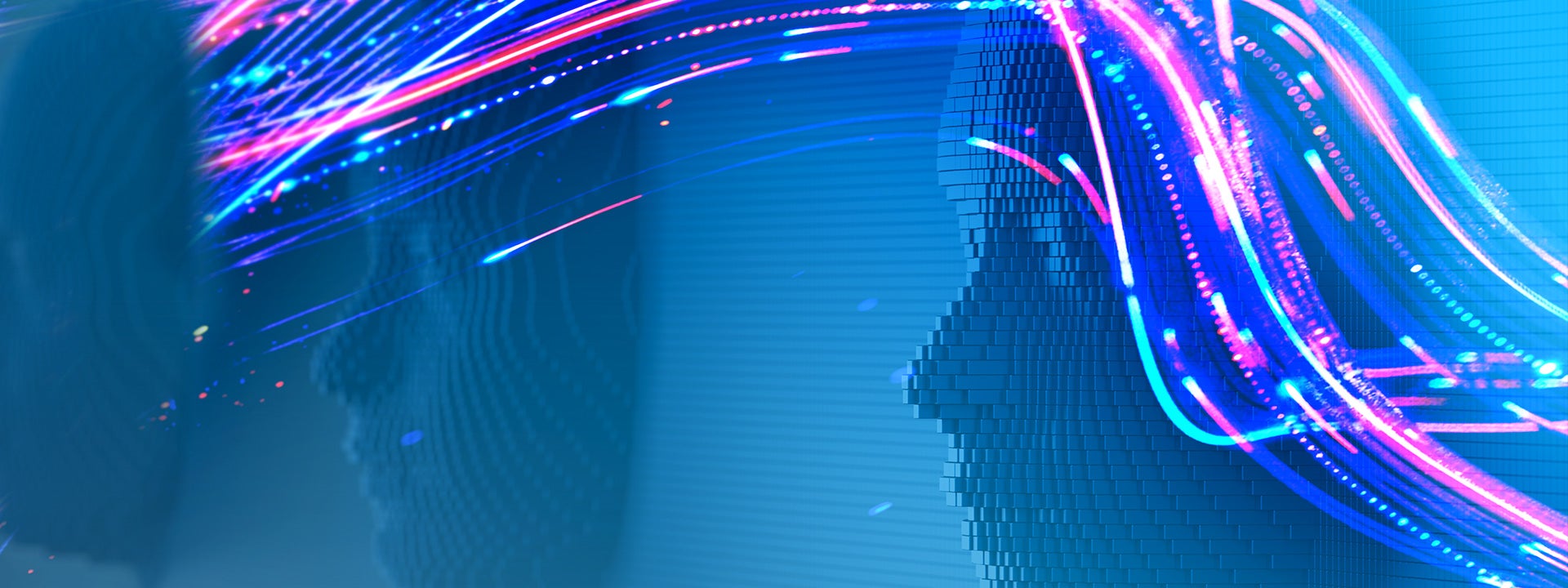
Many corporate risk model management groups don’t have a complete view of all the analytic models in use. Oftentimes, no one really knows how all the data is being sourced to feed those models, or where all those model results go and how they’re being used.
This creates a variety of problems that only become more acute when models go into production, as organizations struggle to monitor model risks beyond the development phase. These issues are exacerbated by tight budgets, limited resources, and the ever-growing appetite for more models.
In these circumstances, companies need to develop better trusted AI governance practices that are explainable and can scale as model development and use grows but also keeps costs down as model volume grows.