Current Situation: A Double Black Swan in Supply and Demand
Amidst the global economic turmoil caused by COVID-19, few industries have been hit as hard as the upstream oil and gas industry. Operators have been knocked off course by the double-black swan events of rapidly eroding demand and a glut of supply.
The global economic slowdown will have a direct impact on general energy consumption, especially in uses such as transportation, industrial manufacturing, and petrochemical manufacturing, as well as all heavy users of crude oil and natural gas. Global demand is expected to drop by ~1% compared to pre-CV19 projections for 2020 demand.
Meanwhile, Russia and Saudi Arabia have each unilaterally maintained or increased flows into an already over-supplied market in early March. In a mix of retaliatory and market share-grabbing decisions, the flow out of the OPEC-Plus nations has resulted in an incremental addition of ~2% of supply to the market. Even worse news for many producers, this supply is coming from well-funded and cheap resources, allowing them to withstand negative or thin margins much longer than most competitors.
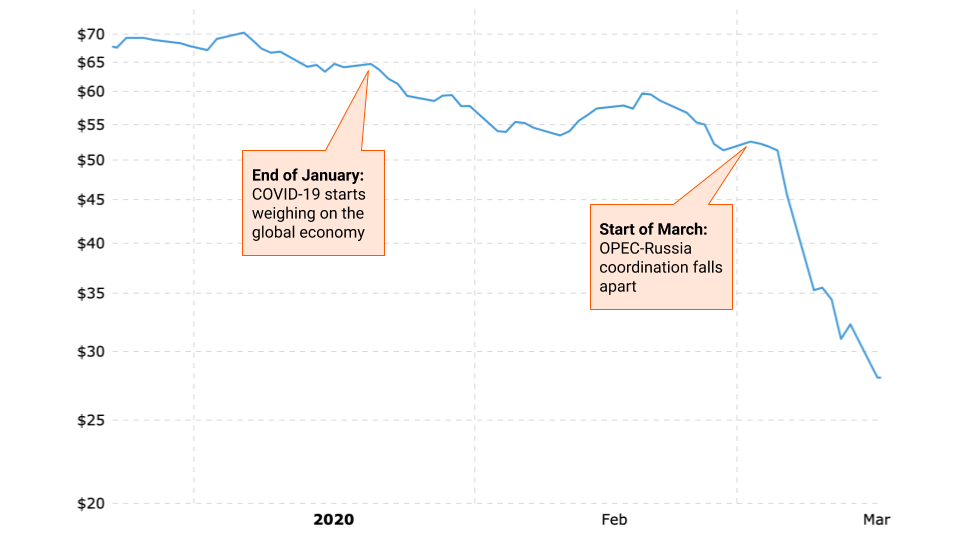
Above: Brent Crude Price Chart (end of Dec. 2019 – mid-March 2020). Source: MacroTrends.net
These two rare and market-shocking events have combined to push crude prices below the marginal cost of production for all but the lowest-cost producers, creating an imperative for new ways of working.
How to Survive: Three Levers for Low-Price Success
Many of DataRobot’s upstream customers have already been employing several common strategies since oil prices reached their peak in October 2018, but have been accelerating their shift to these priorities since the 2020 slide began in January. We’ve consolidated some of the best practices we’ve seen in the industry to demonstrate how producers can ensure that they survive the current tumult and emerge on the other side well-positioned to profit from the eventual commodity cycle upswing.
Levers for Surviving in the New Low-Price Energy Environment
Focus on quality and capital
|
Cash and liquidity will quickly become prime concerns. In the short-term, operators need to ensure they optimize operations to ensure cash flows are sufficient to meet debt service, fixed costs, and other overhead in order to continue as a going concern. In the long-term, they will have to more carefully allocate scarce capital towards only the projects that offer the highest risk-adjusted returns. This will require higher quality in projection valuations, production projections, and reserve estimates, as well as discipline in lease acquisition, project approvals, and capex budgeting. |
Streamline front-line operations
|
Unit economics for each well and barrel are critical to project NPV, so anything that can be done to slide down the cost curve must be employed to get marginal costs below market values. Front-line operations must be lean, effective, and executed with consistency from day-to-day and well-to-well. The best operators have projects that run as finely choreographed ensembles at minimal cost, while others have projects that are executed haphazardly and expensively. Only the former group has the opportunity to survive. |
Economize the back-office
|
Positive cash flow also necessitates discipline and efficiency in the back-office and in key support functions, as these can often add substantial costs to the enterprise and take away from future cash flows to shareholders or capex budgets. Automation and streamlined processes will minimize this erosion of company profits. |
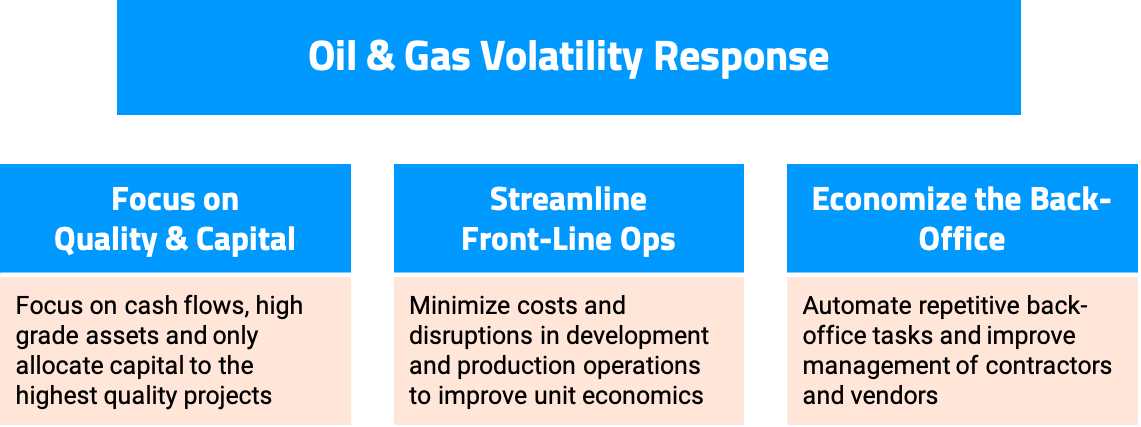
Machine Learning is Critical to Realizing These Strategies
These survival strategies aren’t new and have been employed by most operators to varying degrees of success throughout the history of the industry. However, now more than ever, operators have the opportunity to integrate machine learning into critical operations throughout their organizations to ensure optimal decision-making.
To fully realize these strategies for survival, operators must make smarter decisions on a consistent and scalable basis in real-time.
For example, in order to streamline operations, decision-making that harnesses the knowledge of the entire organization with only seconds to spare needs to be pushed to the wellsite and executed flawlessly in order to prevent negative, costly events (e.g., pump failures, screen-outs, plug slips, etc.)
- Smarter Decisions: decisions, insights, and alerts can be made with consideration of the totality of information and free from bias
- Scalable Decisions: Deploy to more regions, plays, and assets instantly and in a consistent way that is aligned with organizational priorities
- Faster Decisions: Appropriate decisions can be made in real-time
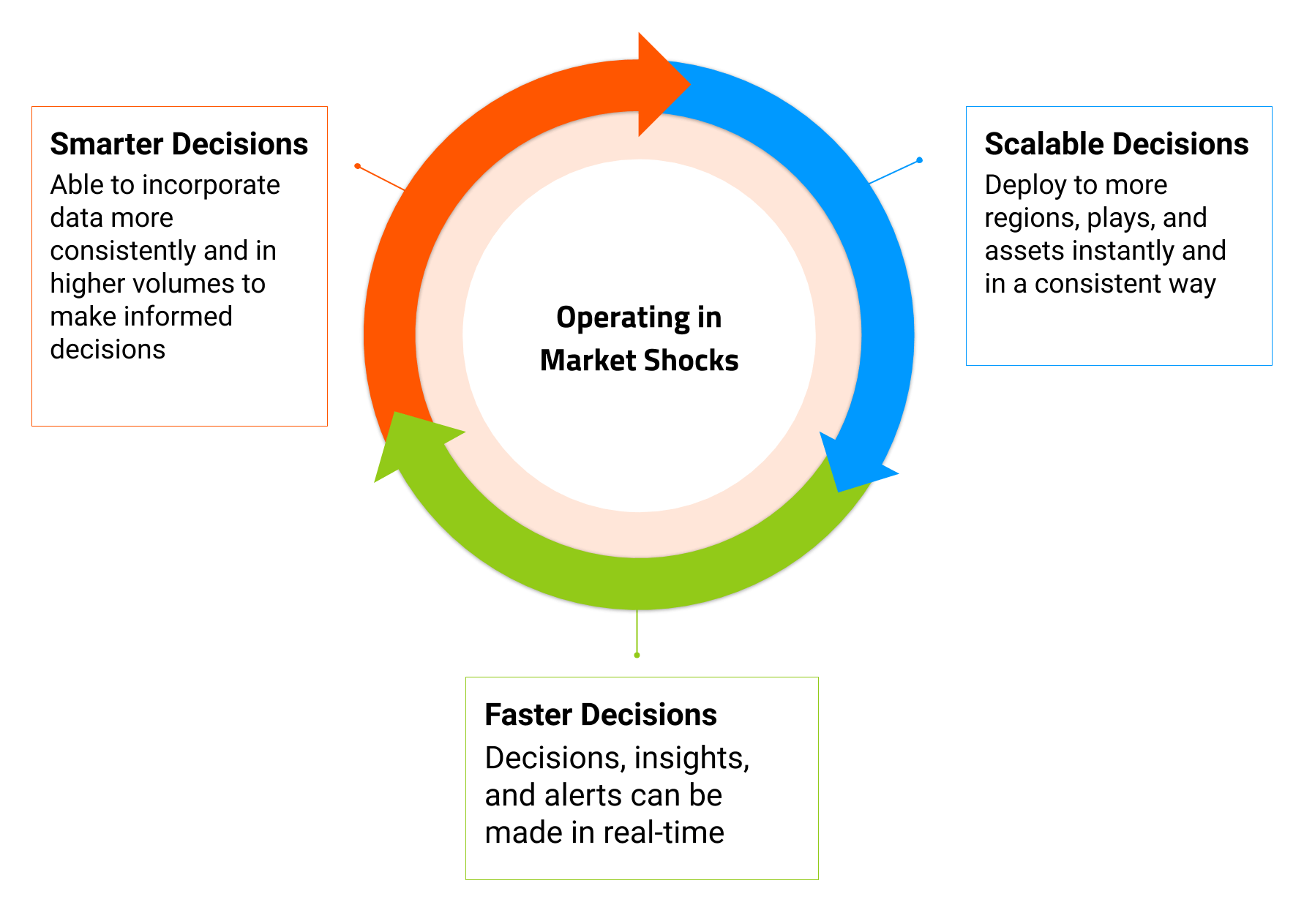
The only way to build operations that realize these requirements for good decisions across the entire enterprise is by embracing machine learning. Machine learning models can codify the right decision-making processes, ensure that they are applied consistently, and make those decisions in real-time.
Getting Tactical: Use Cases to Realize These Strategies
Turning these survival strategies into operational realities requires breaking them down into practical use cases. The table below lists some high-level categories of use cases that operators can explore to define the specific projects that will yield the most benefit for their situation.
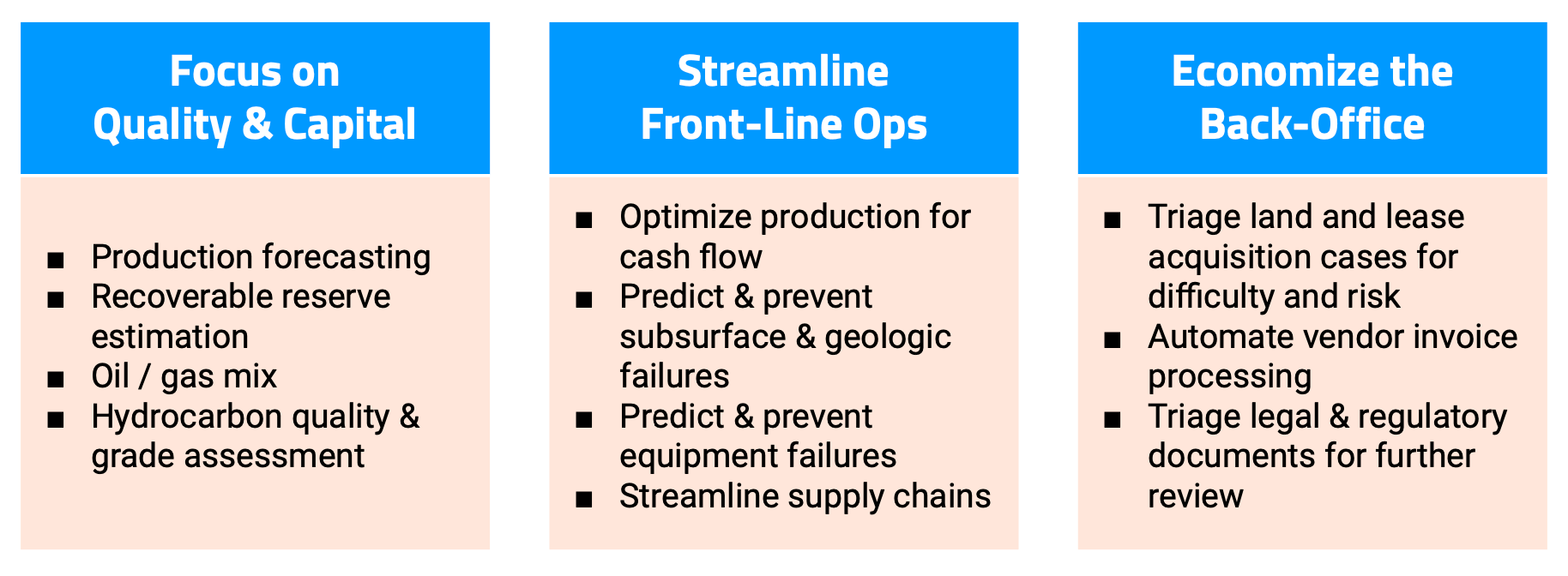
What is important about this current situation is that prioritization of potential use cases must take into account timing and how fast they can yield meaningful cash flow impact. Operators must properly balance projects that put them in a strong position for long-term survival, as well as those that can create short-term boosts in cash flow. We often work with our customers to think about use cases in terms of complexity and avoid the more complex projects. However, in a survival situation, we need to instead think about “Speed to Value,” which includes complexity, but also has a realistic dimension of speed to help with liquidity and working capital management. A generic example of this Value vs. Speed matrix is shown below.
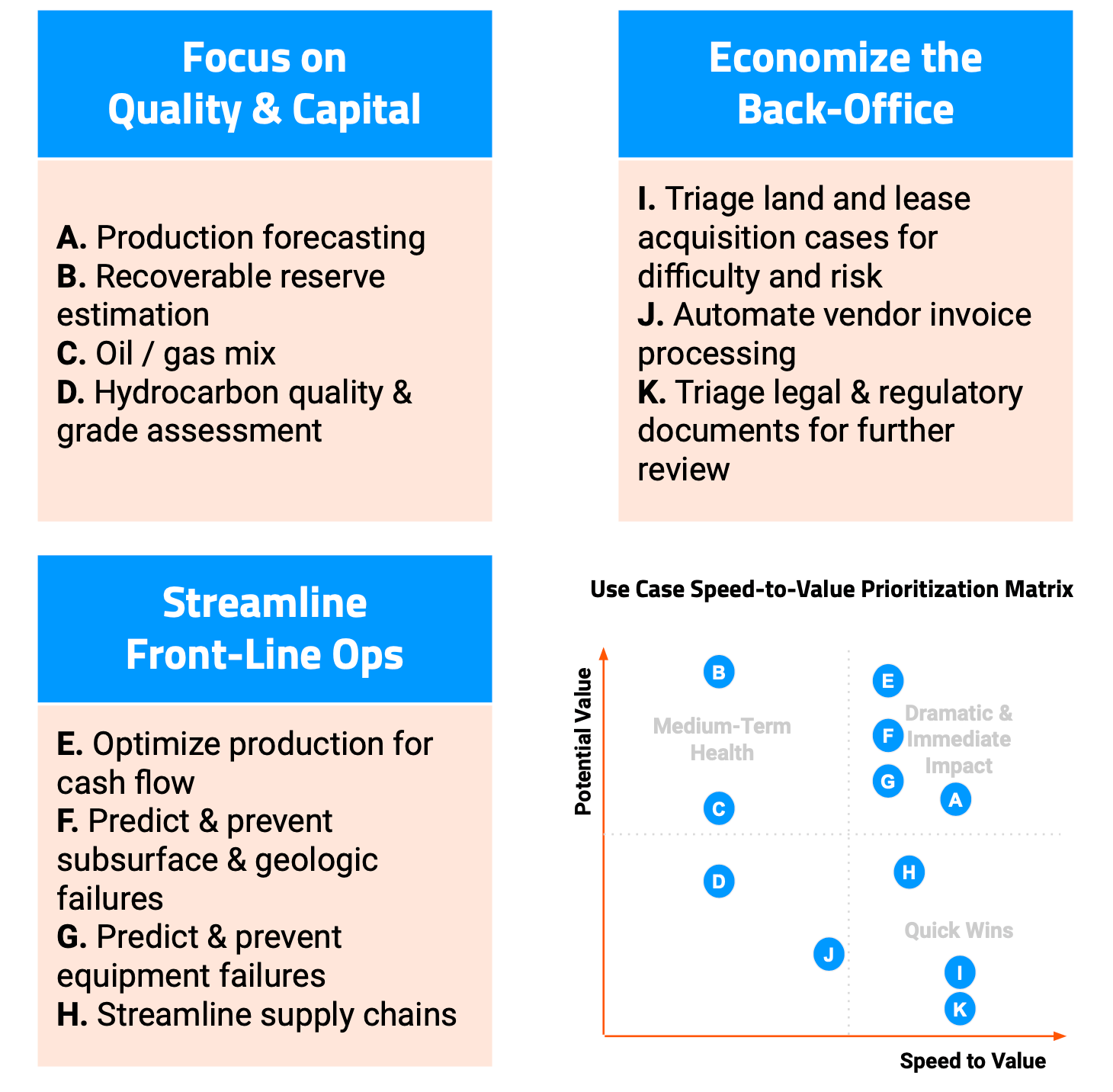
Conclusion
In prior commodity cycle downswings, operators found new ways to push production costs lower and survive. They will do likewise in this one. However, this time, one of the key differences will be the game-changing technologies available to them to make this transformation. In past downturns, those technologies were largely physical: ultra-deep offshore, hydraulic fracking, higher proppant loading, etc. This time, the game-changing technology will be the widespread embrace and exploitation of machine learning, and the first place that will be evident will be in the operators that turn to that technology to survive this current storm.
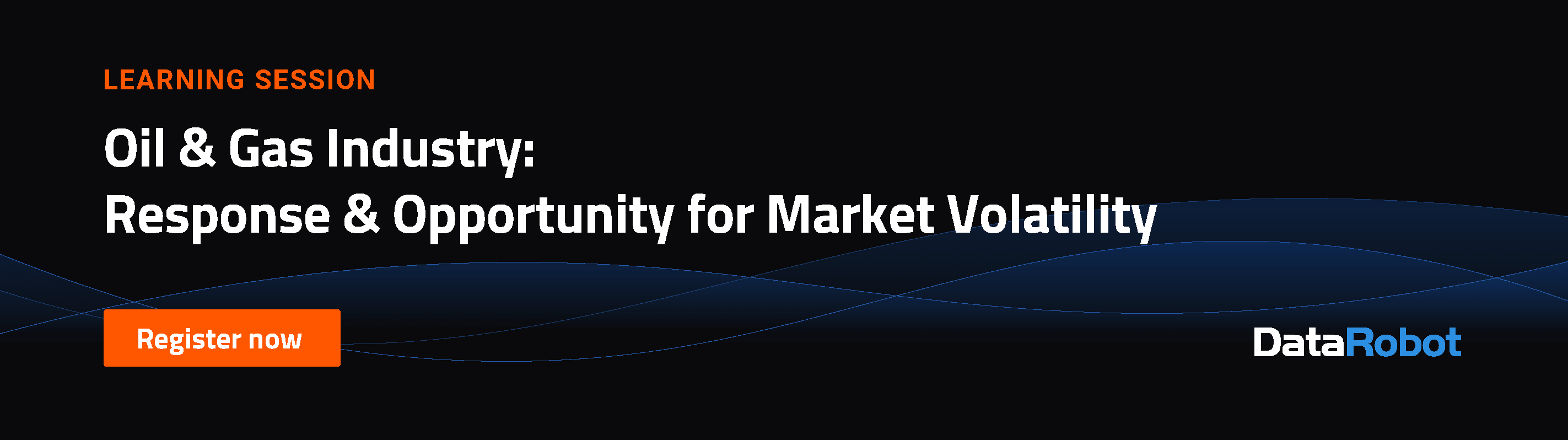
About the author
John Sturdivant
AI Success Director at DataRobot
He has led or advised CEOs in digital transformations across several industries and geographies. He lives in Dallas, TX with his wife and dog. Prior to joining DataRobot, he was Head of Digital and Transformation at TSS, LLC and a consultant at McKinsey & Co.
Meet John Sturdivant