There have been anecdotal complaints that the postseason baseball is deader than its regular-season counterpart, so we used DataRobot to analyze 100k+ batted balls in 2019.
Something is up with baseball this year.
On Wednesday, October 9th, the Los Angeles Dodgers faced the Washington Nationals in an elimination Game 5 of the National League Division Series, and in the bottom of the 9th inning, the game was tied.
Will Smith, a Dodgers rookie, hit a fly ball to deep right field. While it was still sailing, the Dodgers began cheering and leaping over the dugout fence, confident they’d witnessed a game-deciding walkoff home run, and Smith jogged casually towards first base. But Nationals right-fielder Adam Eaton backed up into the warning track where the ball thumped into his glove, just short of the wall. Stunned, the Dodgers players slowed to a stop in the dirt beyond the dugout, expressions turning to dismay.
This hit had not played out as the Dodgers, with their experienced eyes and ears, knew to expect, and it changed the course of the game and the series. But it’d be one thing if this were a single oddball we were talking about. Instead, the regular and postseasons this year have posed a mystery revolving around the heart of the game: the ball and how far it flies.
The “juiced” ball
It starts with a surge. Home run records were shattered this regular season, 11% more home runs than any season before and some of those 6,776 looked really weird, downright funky. Now in the playoffs, we’re witnessing a sudden slump in which teams that saw franchise record-setting seasons are now watching what they expect to be home runs come up short. What’s going on?
Rob Arthur on Baseball Prospectus analyzed the MLB’s pitch tracking data, and posited that there have been 50% less home runs in the postseason than should’ve been and the culprit is increased air resistance on the ball. His theory builds upon work done by Dr. Meredith Wills, an astrophysicist who investigated a surge in home runs in 2017. Dr. Wills deconstructed balls used in the 2014 and 2016-2017 seasons, comparing their individual components, and discovered the laces on the newer balls were 9.0% thicker, resulting in a tighter, smaller ball—and impacting the drag coefficient.
This is the “juiced” ball, altered in order to have higher-scoring, more exciting games; a theory that’s come in and out of baseball circles since the 1990s, but now is gaining more traction again. As a capper to the conspiracy theory, MLB acquired Rawlings, the baseball manufacturer, in 2018 for $395M.
Here at DataRobot, we have our own impassioned fans, some of whom get to incorporate baseball into their professional lives. John Sturdivant, an AI Success Director with DataRobot and amateur baseball sabermetrician, Ari Kaplan, our Director of Industry Marketing and the founder of the Chicago Cubs analytics department, and Andrew Engel, our General Manager of Sports and Gaming, began discussing the mystery of the “juiced” and “de-juiced” ball. John decided, with Ari and Andrew’s help and expertise, to explore what the DataRobot platform and machine learning could bring to light and reveal in this controversy.
Machine Learning Moneyball
Where Rob Arthur looked at pitches, John would look at hits. With machine learning, John’s approach is empirical and accommodates a greater interplay of variables and their relationships than more traditional descriptive analytics. In machine learning, a complex algorithm is trained off historical data—in this case, variables about the pitch, the ball, the stadium—to be able to predict what the outcome—the hit—should be for new records, should the present situation be unchanged from those historical examples. Machine learning therefore can be a powerful tool to simply ask if something has changed.
For this investigation, we used MLB’s StatCast data, which tracks the precise movement of every pitch and hit ball hundreds of times a second to produce rich, quantified descriptions of each play in the MLB season. We also added in contextual data about each pitch (pitcher, hitter, stadium, etc.) to be sure we were capturing everything that could affect ball flight.
Our goal was to determine what postseason batted balls should have looked like based on regular season data, then compare those predictions to actual results. Using DataRobot, John and our team, in less than ten hours, built two sets of models predicting exit velocity off the bat and ball flight distance. We trained them based on data from the 2019 regular season, so when we fed postseason hits into it, we’d learn how far the ball should’ve flown based on the regular season baseball. Any difference between actual ball flight in the postseason and predicted ball flight based on the regular season models would indicate something has changed, like the baseball.
Additionally, to ensure any patterns that emerged weren’t just regular-to-postseason trends (e.g. better players play on playoff teams, so of course the ball will fly differently), John ran this same process for 2018 to see if we could replicate our findings across multiple years. This method would allow us to investigate the ultimate question: Did MLB squeeze the juice out of the 2019 postseason baseball?
The results
The first surprise: the ball is livelier off the bat in the postseason. Launch speed in the 2019 postseason is up 0.53% from what our machine learning algorithm predicts. This is possible in a baseball that has a higher Coefficient-of-Restitution, so more energy from the pitch velocity and swung bat is transferred into the launch of the ball post-contact.
However, hit distance and ball flight is actually down from the regular season. St. Louis’ analytic department has stated the ball this postseason is traveling four-and-a-half feet less than in the regular season. Per our models, the difference is 1.1% less distance on average—plenty enough to see a ball that should land on the far side of the fence die on the warning track instead. This could be an indicator of a less-aerodynamic, higher-drag baseball.
John repeated this same exercise using the 2018 regular and postseasons to test if these trends are simply a result of moving to the playoffs. Using the same procedure, we found no significant difference between the 2018 regular and postseason baseball. Thus far, the change in ball flight characteristics would seem to be unique to 2019.
The MLB has responded to these rumors and denied any modifications to the ball. If this indeed is untrue, we can speculate as to what may have motivated their actions, but whatever they were, it seems to have resulted in a harsh over-correction.
The big question remains, then, if these changes may be drastically altering the dynamics of the postseason. We put out our predictions just a week ago for who will win the World Series, and already the Dodgers, who we narrowly gave the highest probability at 26%, are out of contention. The simplest answer is that we can never know for sure, and the team that triumphs will do so by adapting intelligently as all baseball teams must to a constantly evolving game—which is why after 150 years we’re all still watching, discussing, and hopefully enjoying.
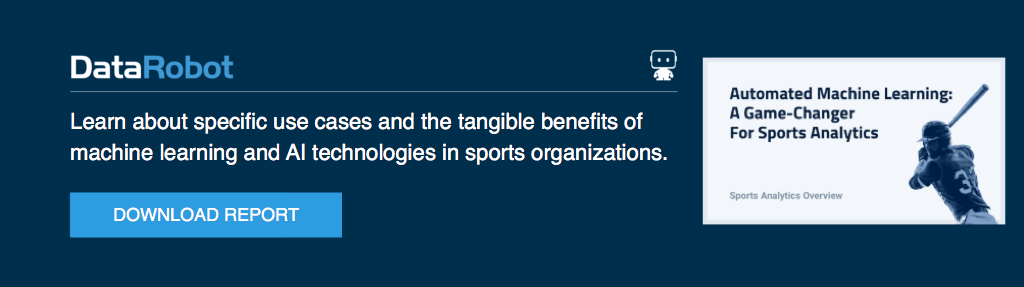
About the author
John Sturdivant
AI Success Director at DataRobot
He has led or advised CEOs in digital transformations across several industries and geographies. He lives in Dallas, TX with his wife and dog. Prior to joining DataRobot, he was Head of Digital and Transformation at TSS, LLC and a consultant at McKinsey & Co.
Meet John Sturdivant
Sarah Khatry
Applied Data Scientist, DataRobot
Sarah is an Applied Data Scientist on the Trusted AI team at DataRobot. Her work focuses on the ethical use of AI, particularly the creation of tools, frameworks, and approaches to support responsible but pragmatic AI stewardship, and the advancement of thought leadership and education on AI ethics.
Meet Sarah Khatry
Andrew Engel
General Manager for Sports and Gaming, DataRobot
Andrew Engel is General Manager for Sports and Gaming at DataRobot. He works with DataRobot customers across sports and casinos, including several Major League Baseball, National Basketball League and National Hockey League teams. He has been working as a data scientist and leading teams of data scientists for over ten years in a wide variety of domains from fraud prediction to marketing analytics. Andrew received his Ph.D. in Systems and Industrial Engineering with a focus on optimization and stochastic modeling. He has worked for Towson University, SAS Institute, the US Navy, Websense (now ForcePoint), Stics, and HP before joining DataRobot in February of 2016.
Meet Andrew Engel