Driving value into the model validation process using automated machine learning
In recent years, the big data revolution has expanded the integration of machine learning models into more and more business processes. It is no surprise that accurate models are a valuable asset to any business. However, due to an increased reliance on models for everyday business processes and decisions, model risk must be managed. This trend has emphasized the importance of model risk management, which in particular has become a hot topic in the regulatory and compliance-rich industry of financial services.
Financial institutions can use automated machine learning to gain efficiency and quickly align their model risk management framework to regulatory expectations.
Financial institutions of all sizes and complexities must face the challenge to meet the increasingly high standards of financial regulators and supervisors. Luckily, there is a technologically powerful solution that institutions can use to gain efficiency and quickly align their model risk management framework to regulatory expectations. That technology is automated machine learning. Financial institutions use automated machine learning to gain efficiency and quickly align their model risk management framework to regulatory expectations.
DataRobot provides the industry’s best integration of automated machine learning technology, utilizing key product features for rapid “out-of-the-box” compliance with model risk management regulations. Traditional model development methods are time-consuming, tedious, and subject to user error and bias. DataRobot uses automation to decrease time-to-deployment for DataRobot models in highly regulated industries such as banking and insurance.
Our focus on automating compliance requirements will increase the overall efficiency of your model development process. However, in addition to increased efficiency of the model development process, efficiency of the model validation process is also meaningfully enhanced using automation, because validation submissions adhere to automated standards before the validation process even begins. This article focuses on two key areas of model risk management compliance automation: model development documentation, and alternative challenger model benchmarking.
Documentation Automation
If a model is insufficiently documented it can delay or possibly prevent the business from gaining value from its predictions. A recent survey conducted by McKinsey & Company found that of the leading financial institutions, 76 percent of respondents identified documentation that is incomplete or of poor quality as the largest barrier for their validation timelines. Clearly, sufficient documentation is one of the most critical elements for effective model risk management, but it is also one of the most time-consuming tasks of model developers.
DataRobot addresses this industry need by investing in product features that will automate the model documentation process required for regulatory compliance. By using automation, DataRobot will reduce the model documentation process that would take several weeks (or sometimes months), down to seconds.
Effective model documentation is so important that the regulation targets it as a key component of an effective model risk management framework. As noted within the Federal Reserve Board’s Supervision and Regulation Letter SR 11-7:
“Without adequate documentation, model risk assessment and management will be ineffective. Documentation of model development and validation should be sufficiently detailed so that parties unfamiliar with a model can understand how the model operates.
Documentation takes time and effort, and model developers and users who know the model well may not appreciate its value. Banks should therefore provide incentives to produce effective and complete model documentation. Model developers should have responsibility during model development for thorough documentation, which should be kept up-to-date as the model and application environment changes.”
By automating components of the model development documentation process, DataRobot standardizes the content within the document to align it with regulatory and institutional policy expectations. The benefits of automated model documentation include:
- Efficiency: Model developers no longer need to create model documentation from scratch. By automating the model documentation process, the development teams free up more time to focus on developing new models and managing existing ones with less time needed for documentation and report writing. This ensures that the requisite amount of documentation is created for model developers, users, and validators to effectively manage model risk.
- Consistency: Automated model documentation ensures that the industry’s best practices are followed. It also ensures that model validation review submissions are consistent and sufficiently detailed, thereby streamlining the model validation review process.
- Speed: A potentially underappreciated aspect of documentation automation is the speed with which documents can be prepared and distributed. With the use of automation, new model documentation can be created in seconds with the click of a button.
The bottom line is that automated model documentation is a powerful tool used to maximize the model development and model validation processes thereby reducing the opportunity for model risk to arise. It maximizes the model development process by giving model developers a head start on the often dreaded and time-consuming model documentation process. This adds efficiency to the model validation process by providing consistent documentation needed for their model review and effective challenge process. The overall impact to your financial institution is a reduction in model risk and an increase in regulatory compliance; not to mention that your Data Scientists will be thrilled to have more time to focus on what they do best: actually performing data science and model development.
Automated Challenger Model Benchmarking
Effective challenge is a leading principal of model risk management regulation, but the burden to ensure effective challenge does not lay solely on model validators. The model developer must also perform certain tasks during the model development process to ensure the model is appropriate for its intended use. One such task is creating alternative challenger models and comparing the performance of these models with the “champion” model.
As stated by Supervision and Regulation Letter SR 11-7, “developers should ensure that the components work as intended, are appropriate for the intended business purpose, and are conceptually sound and mathematically and statistically correct. Comparison with alternative theories and approaches is a fundamental component of a sound modeling process.”
Challenger model benchmarking and comparison is a fundamental component of a sound modeling process that is expected by regulators. DataRobot uses automated machine learning to automatically develop, test, and benchmark scores of alternative models in a matter of seconds, rather than the weeks or months it can take using manual processes. Dozens of challenger models are posted to a leaderboard so that users can easily compare and benchmark different models, then select the most appropriate one for the business problem being addressed, in accordance with regulatory expectations.
The progress of the alternative models’ performance is captured by DataRobot’s Leaderboard, which actively ranks each model based on its accuracy (see Figure 1 below). Therefore, during the modeling process, DataRobot develops dozens of independent challenger models, and exposes the details of how these models were built and how they perform, which enables the user to select the best model for the particular business problem being addressed, in accordance with regulatory expectations.
Figure 1: DataRobot Leaderboard for a Probability of Default modeling using publicly available data from LendingClub.com.
Alternative models play an important role during model development. They allow model developers to show effective challenge during the model development process by providing empirical support for the final champion model. In this way, DataRobot provides a unique framework for model developers to test and benchmark cutting edge machine learning models.
Conclusion
DataRobot’s automated machine learning platform offers a much more robust framework for model risk management than traditional manual modeling, and we are leading the industry in using automated machine learning to minimize model risk. We deliver the tools to optimize and accelerate model risk management, making it easier for banks of all sizes to gain value from a robust model risk management framework.
More on this topic:
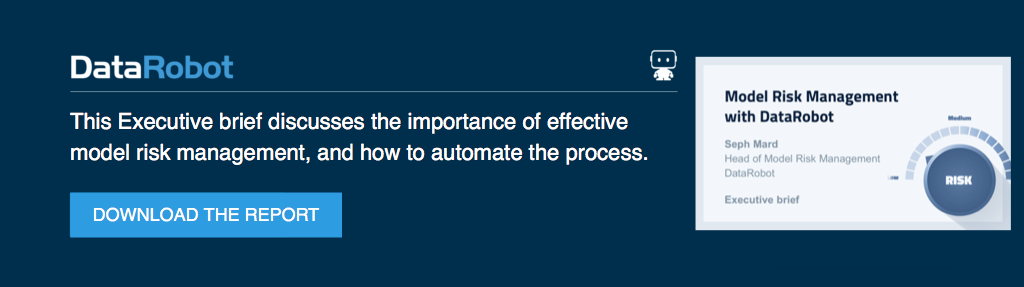
About the Author
As the head of Model Risk Management at DataRobot, Seph Mard is responsible for model risk management, model validation, and model governance products, as well as services. Seph is leading the initiative to bring AI-driven solutions into the model risk management industry by leveraging DataRobot’s superior automated machine learning technology and product offering. Seph has more than 10 years of experience working across different banking and risk management teams and organizations. He started his career as a behavioral economist with a focus on modeling microeconomic choices under uncertainty and risk, then transitioned into the financial services industry with a focus on model risk management and model validation. Seph is a subject matter expert in model risk management and model validation.
About the author
Seph Mard
Head of Model Risk, Director of Technical Product Management, DataRobot
As the head of Model Risk Management at DataRobot, Seph Mard is responsible for model risk management, model validation, and model governance product management and strategy, as well as services. Seph is leading the initiative to bring AI-driven solutions into the model risk management industry by leveraging DataRobot’s superior automated machine learning technology and product offering.
Meet Seph Mard