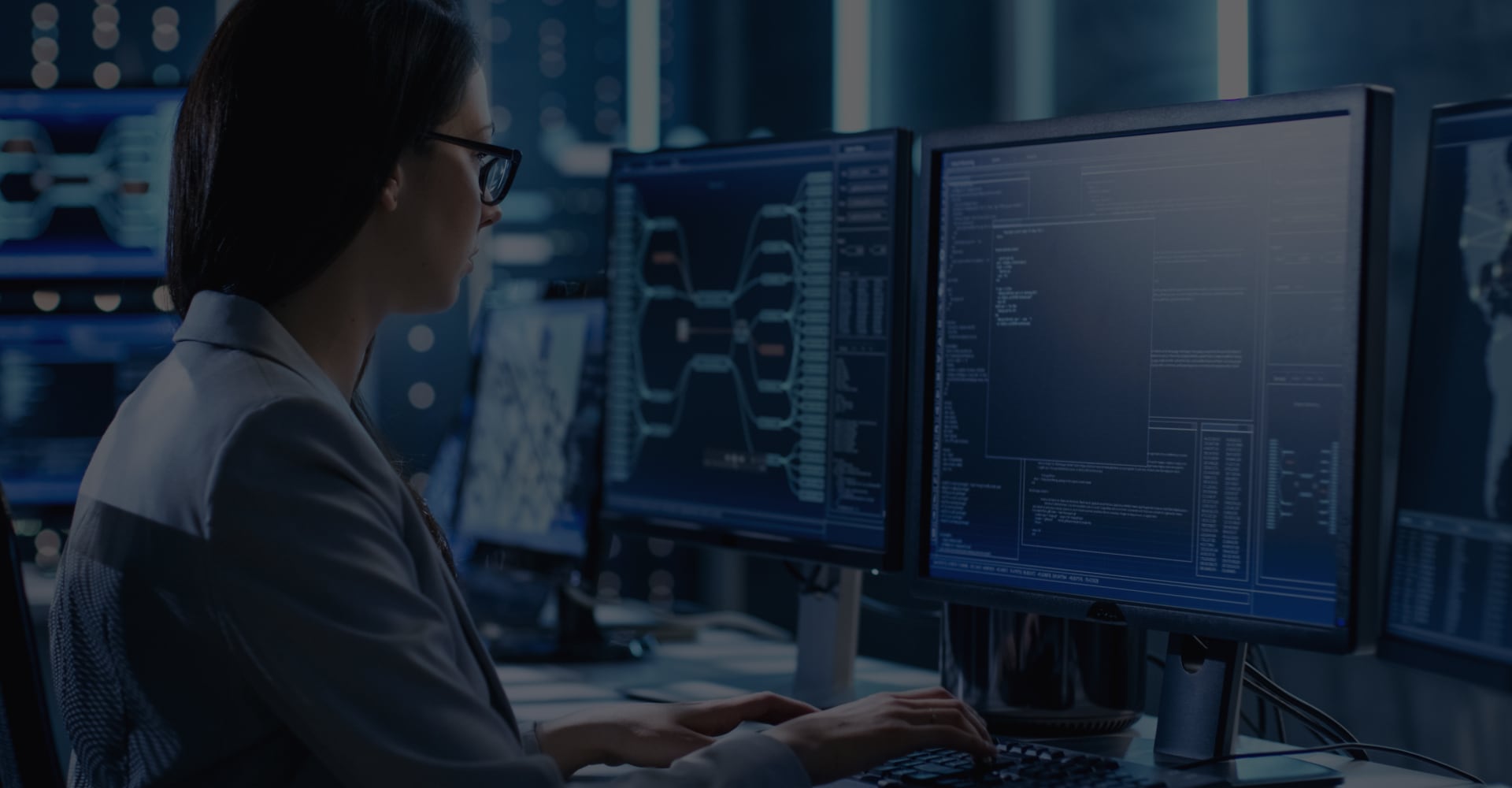
Governance and Monitoring as Dimensions of Trusted AI
The best-designed model, with poor governance, might still result in undesired and unintended behavior. Find out how to build in good governance and monitoring to ensure your AI system delivers the value you need in production.
Model Governance Is a Core Ingredient of Trustworthy AI
The best designed model, with poor governance, may still result in undesired and unintended behavior. Governance refers to the human-machine infrastructure that oversees the development and operation of a machine learning model. Dimensions of trust like compliance, security, and humility may potentially fall under the purview of your governance structure. While the exact requirements of good governance vary from model to model, depending on the application and intended use, in general, it is critical that a clear system of monitoring, accountability, and redundancy be in place.
What Does Good Governance of an AI Model Require?
Monitoring, traceability, and version control are absolutely necessary for the attribution of any errors or incidents in your system. Well-maintained documentation will also make it easier when it comes time, as is inevitable with machine learning systems, to retrain the model or update the process.
Model monitoring in production can track multiple dimensions of trust. As with evaluating the initial model, a multidimensional perspective on accuracy, ongoing assessments of incoming scoring data, and a record of predictions that can be monitored for instability are all relevant considerations for the ongoing performance of the model. Any appropriate humble trigger conditions should also be logged. Bias and fairness metrics, when needed, and model explainability tools round out a complete and comprehensive set of dimensions related to the model itself. The production environment is also a matter of concern, tracking any system errors and uptime.
What Can Go Wrong with an AI Model in Production?
Machine learning models are built on a snapshot in time. The relationships observed between your predictive target and the features in your training data are likely to evolve and lose relevance. This is expected behavior, and its rapidity depends principally on the rate at which your underlying business process changes.
Monitoring accuracy and a quantity known as data drift can assist you in identifying when it is time to retrain a model on a more recent subset of data. Data drift measures the differences between distributions of your training features from those features observed in your scoring data via a metric known as the population stability index.
Changes can also be sudden, however. When black-swan events occur, a model that was completely performant the day before might no longer capture at all the dynamics of the new situation. In another scenario, an outage or system issue might remove your ability to access the production environment, machine learning model, or its predictions. In these instances, backups and redundancies are critical.
A redundant system to the model could be a mirror of the original model, stored on another server, or it could be a computationally simpler model or set of business rules that can govern your process safely in the model’s stead. In the event of failure, for some applications it might also be appropriate to have a manual process in place that can replace the model’s previous operation, even for a time.
Who Should Be Responsible in My Organization for the Performance of an AI Model?
The ongoing trustworthy performance of an AI model likely requires the joint oversight and collaboration of the following roles:
- Information technology specialists
- Data scientists
- Business users
Governance and Monitoring Are Just Pieces of the Puzzle
Governance and Monitoring that harmonize the use of AI with your business as a whole are just one of the dimensions of trustworthy AI operations. The full list includes the following:
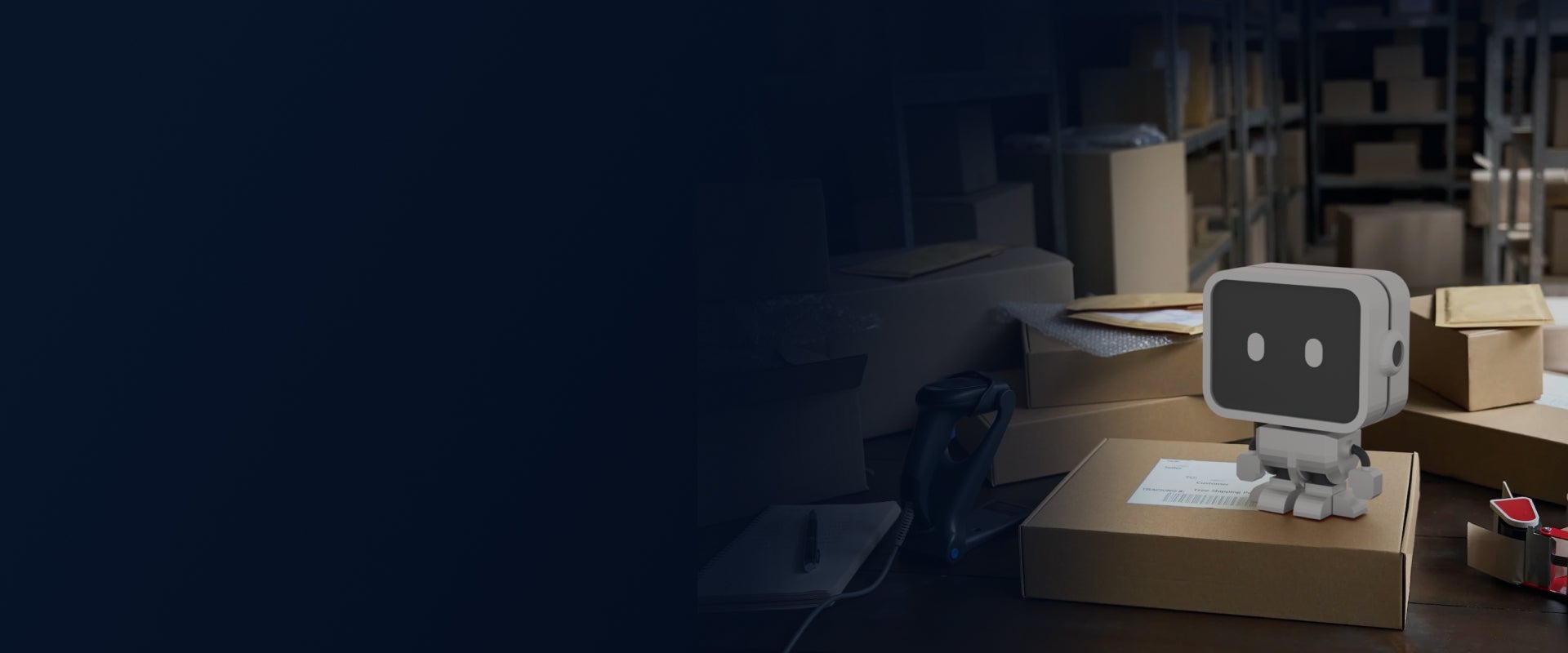