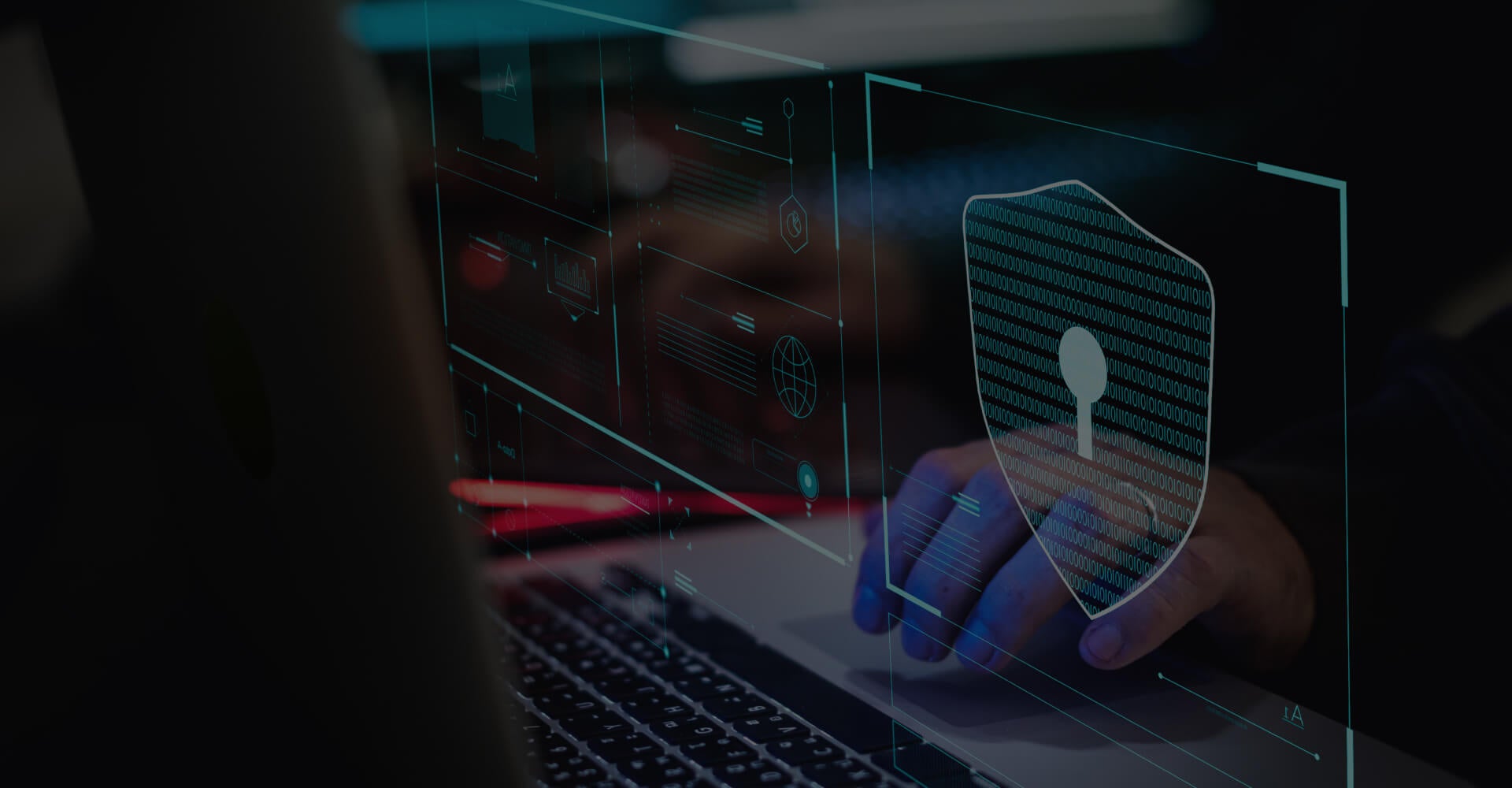
Security as a Dimension of Trusted AI
Sensitive information for your enterprise, such as revenue numbers, employee performance, salary, personal data, and sales leads, might be part of your training data. Find out how to ensure your data and model stay secure.
Sensitive Information Is Integral to the AI Lifecycle
There are multiple domains of sensitive information potentially applicable to an AI or machine learning modeling project. Sensitive information for your enterprise, such as revenue numbers, employee performance, salary or personal data, and sales leads, might be part of your training data. Other models might be built on potentially sensitive client data. The predictions of the model might also be sensitive, either due to the nature of the information they reveal or how they impact your decision-making. Finally, the operation of the model itself might be proprietary and of significant importance to keep secure to prevent abuse or manipulation.
What Data Used in or Created by an AI Model Might I Want to Keep Secure?
A model pipeline might share a similar path to implementation as more standard software applications, progressing through development, staging, and production environments. Within and moving between each environment, sensitive information must be kept secure, particularly when transmitted. Development might be the riskiest juncture for the potential mishandling of information as raw data is collected, cleaned, and shared to ultimately train and validate the model. That raw data is more likely to contain private or personally identifiable information (PII) on your customers or employees. For more information on how to handle privacy in particular, see here.
What Are the Characteristics of a Secure System?
Independent and international standards, such as ISO 27001, exist to verify an information security management system’s operation. For another example, SOC 2 Type II certification verifies that a system will keep client or customer sensitive data secure.
For more information on DataRobot’s InfoSec certifications and privacy standards, see here.
What Level of Transparency Do I Want to Let Users Have Access to Regarding the AI Model?
Transparency can be described along a spectrum and carries different security concerns. At the most extreme end, the model functions as a black box, in which the user inputs information and receives a prediction, with no insight into how the prediction was reached. On the other end, maximum model transparency or white-box models potentially expose the entire architecture of the AI or machine learning model, up to and including parameters, data, and code. However, there is a stark difference between transparency and explainability, which we explain here.
In between pure white- and black-box models, you have the choice to share information such as prediction intervals, which quantify the confidence of the prediction, or prediction explanations, which surface the major drivers of an individual prediction. Even this information can potentially expose some of the mechanisms of an otherwise secure model, though it also facilitates the user’s trust in and ability to interpret a prediction. Prediction intervals, in particular, have been shown in research to be potentially leverageable in adversarial attacks on a model hosted on a public API. These are trade-offs that you must knowingly and conscientiously navigate when determining how much information to disclose to users.
Security Is Just a Piece of the Puzzle
Security that harmonizes the use of AI with your business as a whole is just one of the dimensions of trustworthy AI operations. The full list includes the following:
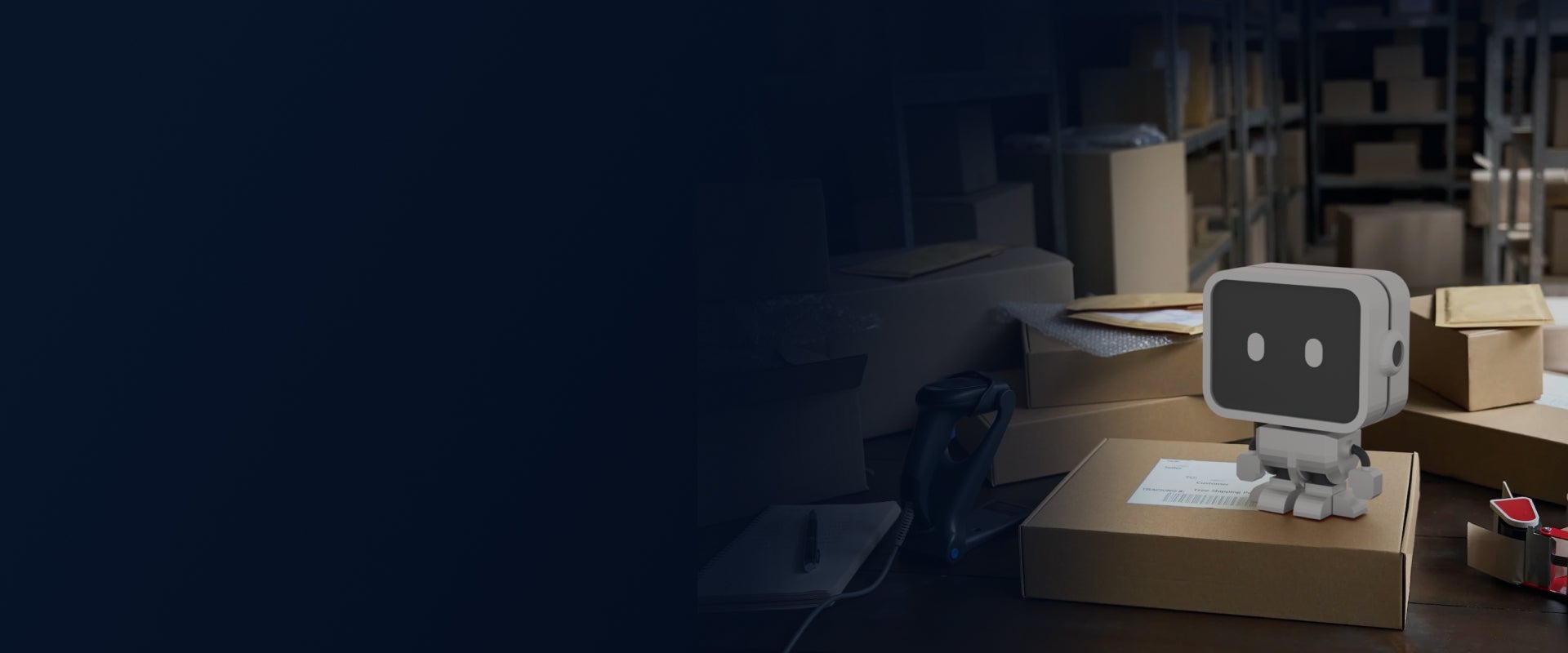