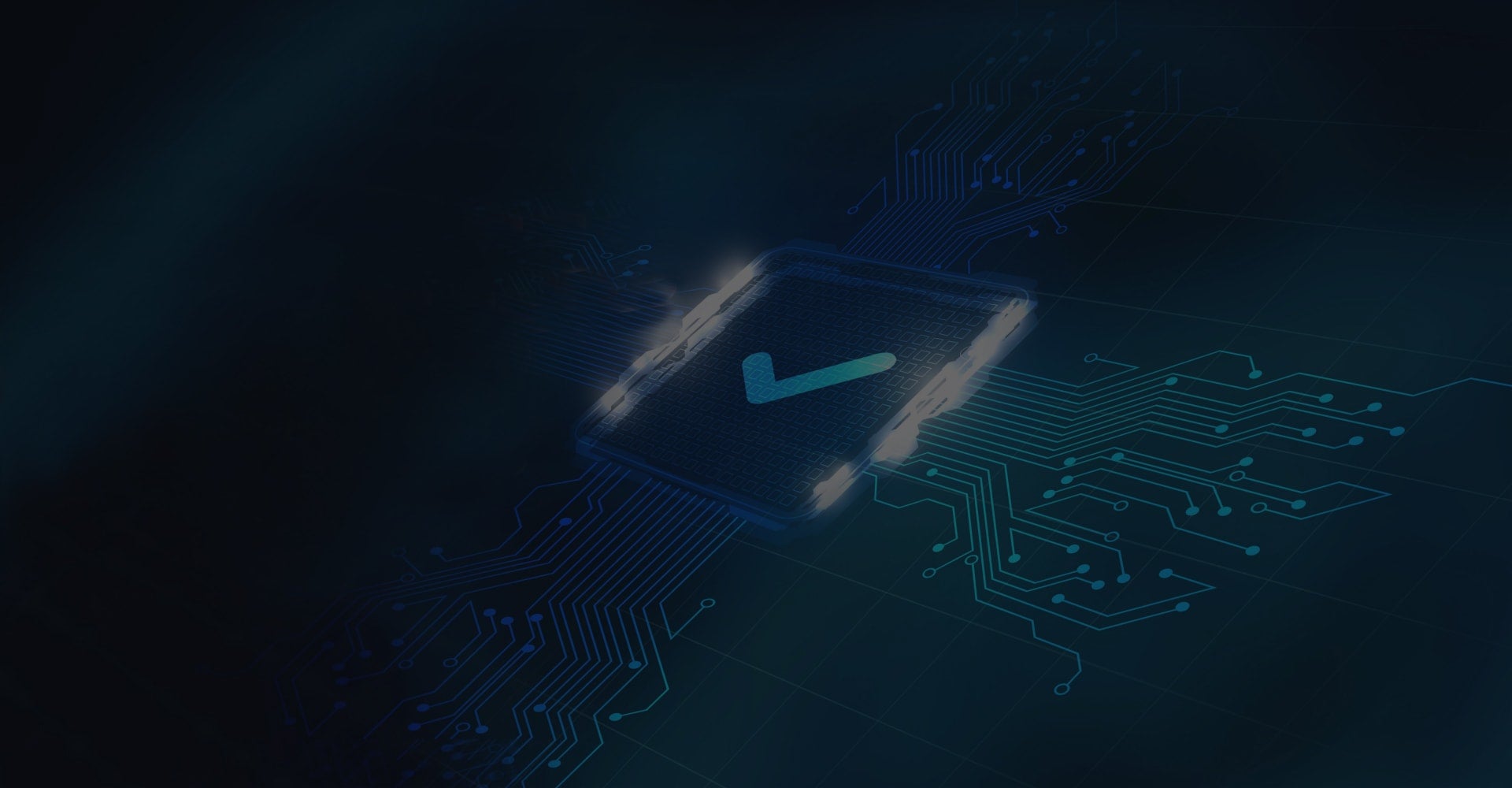
Compliance as a Dimension of Trusted AI
Depending on your industry, aligning model performance to regulatory requirements may be an essential step when preparing to put a model into production. Find out how to set up your model for successful review.
Compliance Is Not a Niche Concept in AI
Applications like banking and credit, insurance, healthcare and biomedicine, hiring and employment, and housing are all subject to significant regulation and scrutiny. That said, even models used in digital advertising can have specific requirements for regulatory compliance. Let’s take a closer look at some of the pressing questions around compliance in AI.
Is Model Compliance Something I Need to Worry About?
This is likely your first question and one best referred to your legal team. The model development process should be informed by a cross-departmental team of stakeholders, from legal to InfoSec to your end business user. This will help facilitate the most comprehensive understanding of a model’s needs and impacts, and thus ensure that the model ultimately fulfills its desired value. Potential risk and hazard identification and mitigation should be one of the first areas addressed when initiating an AI project, and they should inform decisions made at each juncture of the modeling process.
What Might Be Required to Make Sure a Model Is Compliant with Industry Regulations?
Although this is likewise best informed by knowledgeable stakeholders in your enterprise, there are generally three domains where model risk management and regulatory compliance must be established:
- Model development
- Model implementation
- Model use
Even an appropriately designed model can be misused in implementation, as predictions are consumed. Risk management will seek an understanding of what ongoing monitoring and risk mitigation procedures accompany the use of the model.
Within model development, it may be necessary to supply documentation regarding the data provenance and characteristics, to evaluate what inputs are used in the model and how. The results of model validation are likely also of significant interest. It may be necessary to establish that the methods used to validate the model were comprehensive and thorough; as discussed in Performance, no single error metric is likely to provide a strong enough argument. In applicable situations, it may also be necessary to report on model bias and fairness, such as disparate impact.
What Can I Do to Make the Compliance Process as Smooth as Possible?
Robust documentation throughout the end-to-end modeling workflow is one of the strongest enablers of compliance. Best practices of version control and traceability also aid the model development process. The use of explainability tools and appropriate model transparency are major facilitators of describing and interpreting the model’s operations. An impact assessment, conducted with the oversight of an appropriate group of stakeholders, will clarify the model’s ability to satisfy its explicit purpose and assist in the identification of risks, both in the modeling process and downstream in its use and implementation.
DataRobot automatically generates comprehensive and customizable compliance documentation on any of its modeling approaches uniquely applied to your data.
Compliance Is Just a Piece of the Puzzle
Compliance that harmonizes the use of AI with your business as a whole is just one of the dimensions of trustworthy AI operations. The full list also includes the following:
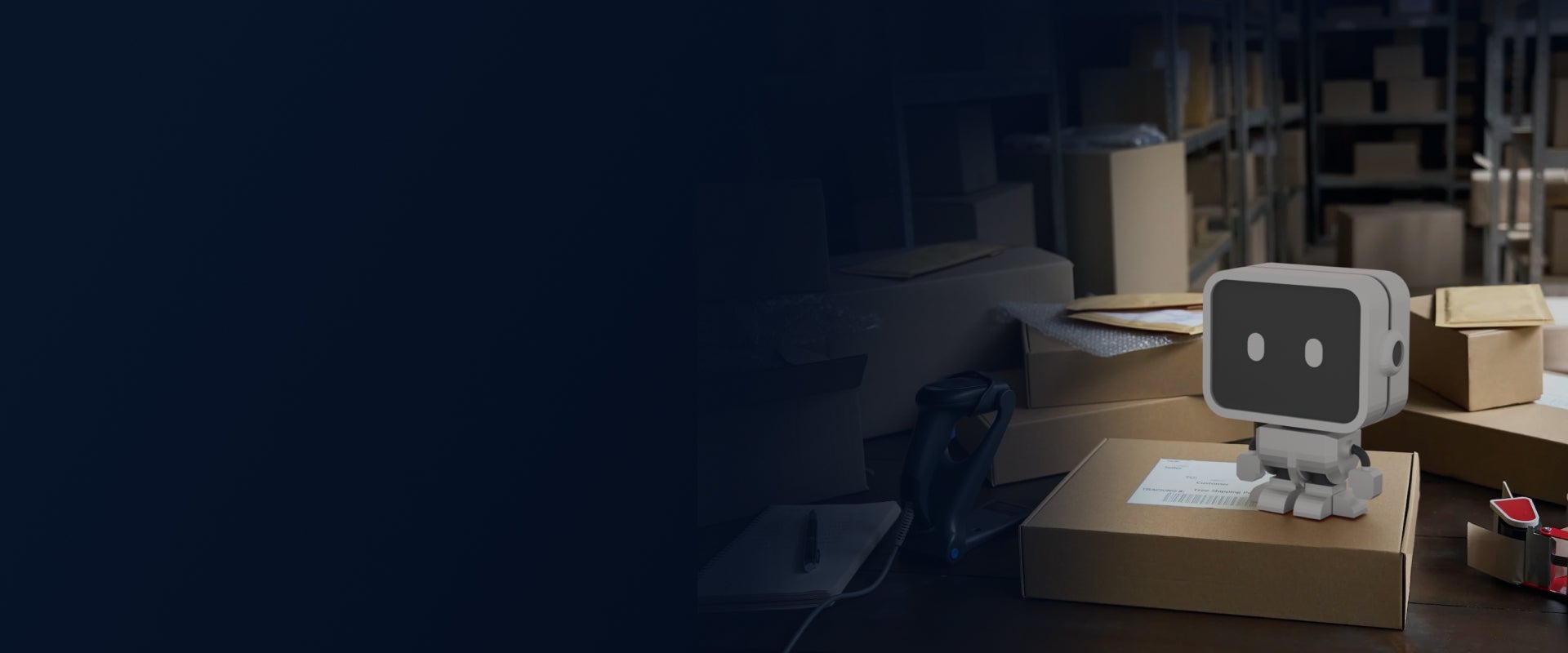