A few years ago I sat in on a presentation by Stephen J. Dubner, one of the authors of “Freakonomics” and host of the “Freakonomics” podcast. Mr. Dubner was giving a talk on thinking analytically, and explaining how answers to current problems may come from someone not directly involved in solving the problem at hand.
The example he gave was about the biggest problem plaguing cities (particularly New York City) in the early 1900s — horse droppings. In the days when the primary mode of transportation was horse-drawn buggies, the biggest municipal issue was how to get rid of the never-ending mounds of horse droppings that piled up all over the city streets. The problem got so bad that it was the highest priority issue for city hall, which had their best and brightest minds working on it. Cottage industries had sprung up just to help manage the tons of horse droppings that were being produced.

Although not specifically designed to alleviate the horse dropping problem, the invention of the automobile and other mechanical modes of transportation eventually made the problem obsolete. This story highlights how, throughout history, automation has had significant impact far beyond the problem it was solving.
Here is another example of the impact of automation on society. Johannes Gutenberg invented movable type with the printing press to make his job easier. What the printing press did, however, was initiate the spread of knowledge to the common man, which eventually led to the Reformation and the transformation of religious thinking and practice.
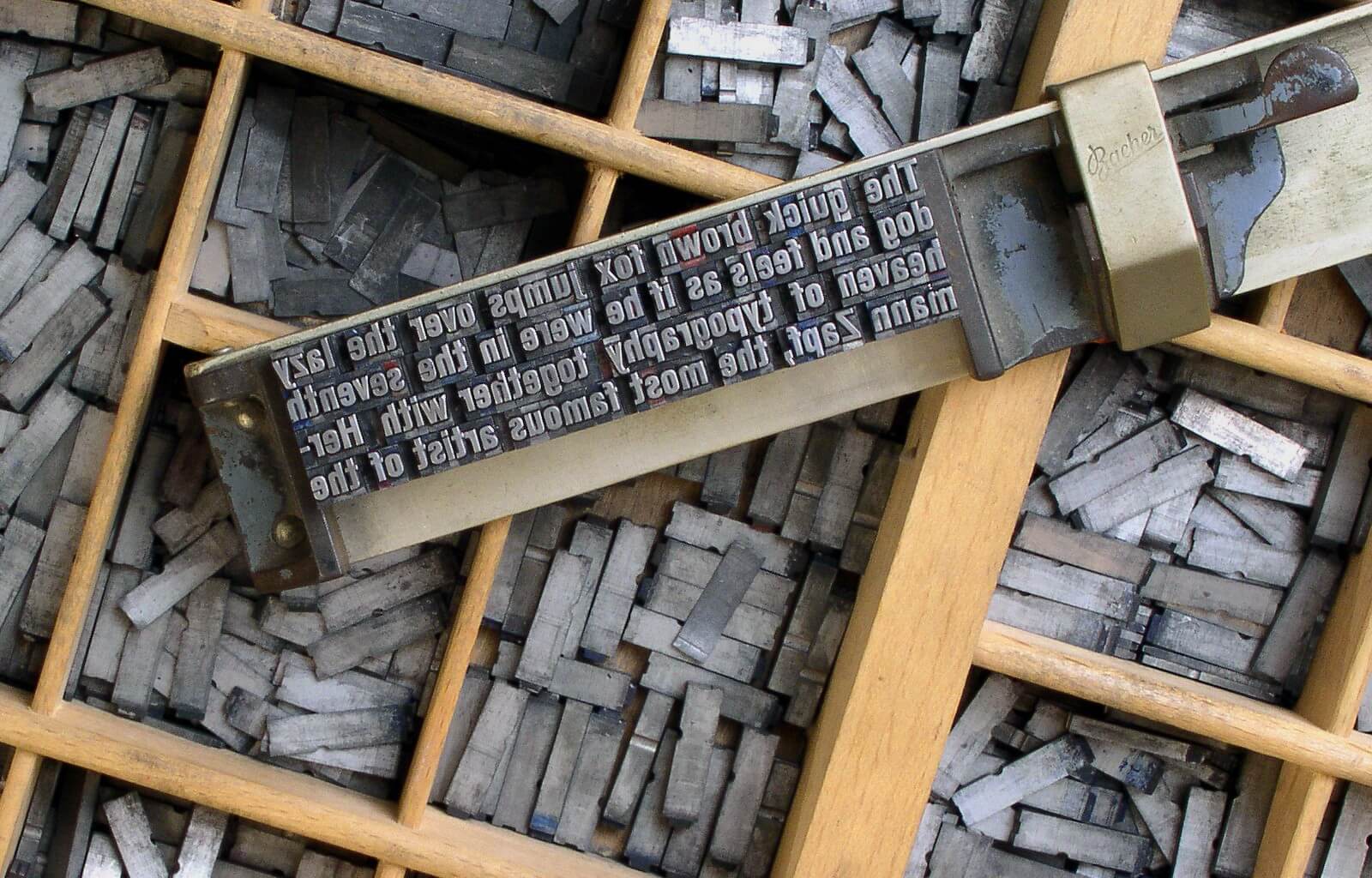
Bringing a Knife to a Gunfight
In today’s economy, companies are struggling with the mounds of data that they are collecting and storing, just as New York City was struggling to keep up with the mounds of horse droppings at the turn of the century. Data collection and storage has been automated in Hadoop appliances and data warehouses, but trying to make sense of the insights hidden in that data makes data scientists and analysts feel like they are being buried under a mountain of horse (expletive deleted).
For many companies, it seems like the solution is to hire more data scientists, teach others to become data scientists, or provide better tools for data scientists to collaborate. As a data scientist myself, I understand the value that data scientists bring to an organization. But, the pace of data creation makes it feel like we’re bringing a knife to gunfight.
Data is growing too fast. Bigger shovels and more bodies are not the answer. If the data being generated is collected automatically, we need to think about applying automation to make sense of the data. We need to apply automated machine learning to deliver insights and provide predictions that drive real business value.
.
Getting Past The AI Hype
As with any emerging technology, automated machine learning often seems more about the hype than the product itself. Consequently, when talking to data scientists, I can often sense the skepticism when discussing automating machine learning. They are keenly aware of how difficult this is.
True automated machine learning should automate the complete data science workflow as much as possible, starting from initial feature engineering and preprocessing, discovering the most optimal workflows and algorithms with automated insights, and ultimately allowing the user to easily deploy the model into production with minimal technical overhead.
Some companies have focused on making machine learning easy by removing the need to code and letting users simply create data science workflows, which involves dragging and dropping various preprocessing, validation, and model training steps. A big flaw with this approach is that it requires the users to know what to drop where and why.
Others have focused on providing algorithms that appeal to experienced data scientists where a bulk of the automation is centered around automated hyper parameter tuning.
True automated machine learning should automate the complete data science workflow as much as possible, starting from initial feature engineering and preprocessing, discovering the most optimal workflows and algorithms with automated insights, and ultimately allowing the user to easily deploy the model into production with minimal technical overhead. This is no doubt ambitious, but it is our vision at DataRobot.
Instead of focusing on bigger shovels, our vision is to truly revolutionize the current state of artificial intelligence with automated intelligence.
In fact, after giving a demo of the DataRobot automated machine learning platform to a team of data scientists at a large company in Seattle, the Director of Analytics stopped me on the way out the door. He had done some research online and brought us in for a demo to compare DataRobot against a platform he already had in mind, mostly to “check the box” as he built a case for purchasing the other platform
After seeing our demo, he told me he was actually surprised. His team had looked at a number of solutions that talked about automation, but DataRobot was the only one that actually demonstrated it!
I find this reaction to be pretty common. With so much marketing hype around automated data science, many data scientists and analysts are skeptical – or jaded – having seen ‘automated’ machine learning platforms with very limited degrees of actual automation. They are unaware of what automated machine learning really looks like, or in some cases, they have been misled by vendors who are hyping up their limited capabilities.
I’ve been in this industry a long time and have worked at various startups that have tried to bring machine learning platforms to market. One thing I know is that my friends and former colleagues at these other companies understand that DataRobot is doing something completely different and incredibly cool. Instead of focusing on bigger shovels, our vision is to truly revolutionize the current state of artificial intelligence with automated intelligence.
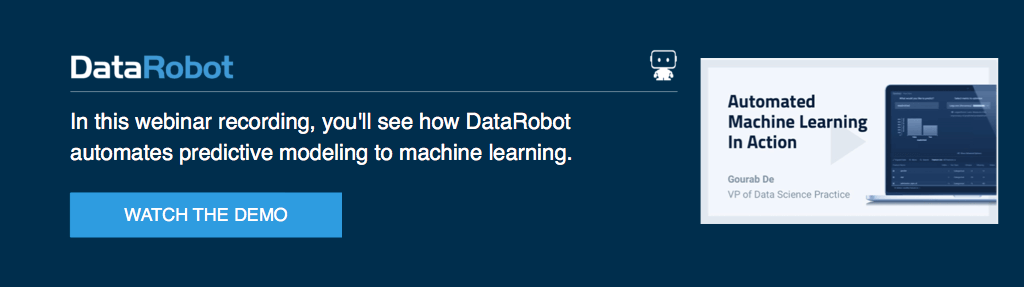
About the Author
Yong Kim currently works as a Customer Facing Data Scientist at DataRobot, where he leverages his wide breadth of experience in defining and solving real-world business problems with data science for enterprises across industries like healthcare, finance, marketing, and software development. Prior to starting at DataRobot in early 2016, Kim held a variety of big data and machine learning solution-oriented roles at companies like Apigee, Predixion Software, and DataQuick Information Systems. He has a proven aptitude for articulating the business value of complex analytics projects and holds a patent for a data analysis and predictive modeling method, as well as a pending patent for his home pricing model validation method.