This post is for leaders that continually hear about data science and machine learning and plan on bringing this technology to their company, but don’t know where to start. By the time you finish reading this article, you will learn how simple it is for you to get your company initiated on machine learning and bring yourselves new and tangible value.
Every technology conference you attend these days, almost every meeting at your company, and in every innovation tournament conducted, you see some aspect of data science. You are a manager that supports new technologies, and you are quite eager to be an adopter of new technology. You want to be a leader when it comes to bringing new and undiscovered value to the company that you work for.
However, you have two important challenges:
- You don’t know where to start on data science
- You are unsure just how difficult it might be to implement data science at your company
No need to worry! Below are a few simple, yet very effective, guidelines that will help you address these two challenges.
Knowledge and Action – Knowing just a little of what data science is all about and then acting on that knowledge is all it takes for you and your team to get impressive results.
The Knowledge Phase
In this blog post, you will find me referring to data science and machine learning analogously. In a nutshell, machine learning models help you predict which marketing channels will bring you the most customers, which loans might default, when a patient might return to the hospital with the same condition, and so on.
Data science, as the name suggests, has two parts to it that are highly essential – data, and the science of making use of that data.
Get some Data:
Without the data, there is no data science.
There are two types of data: internal and external. As you are just getting started, it is wise to start with internal data as you already have it and it is much easier to access compared to external data. Internal data includes your transactional data, master data, historical data, client data, sales data, marketing data, and so on.
Sign up with an Automated Machine Learning tool:
Tools such as DataRobot save you the agony of having to hire data scientists, finding the best data scientist, paying them very high salaries, and then not knowing whether or not they are any good for three to six months. Any business or data analyst that you already have in your company can be very effective using an automated machine learning tool.
The Action Phase
Make it Iterative:
One common pattern that we find in many successful processes such as Agile or Lean Startup is that they are all iterative in nature. You take small steps, but many of them, so that you get an opportunity to correct missteps along the way.
When procuring data (internal data in your case) don’t create panic in your technologist by telling him or her that you need to build a massive data lake using Hadoop or some fancy appliance. Ask for just a little of the internal data to begin with.
Put your data into DataRobot (or whichever machine learning tool your purchase) and see what results it will produce. Not happy with the results? No problem. Call DataRobot for help, or even better, try to figure out why your results were not as good as you had expected. In my experience, your first model will still be better than no model at all.
Aim for Small Wins:
People are attracted to success. Show the first model you have built to your team, to the data creators, the database admins, and those higher up in your organization. Let them see how their contributions have helped.
Write down a list of Hypotheses:
I once informed one of our automated machine learning product users that data science is all about writing down a list of hypotheses and then testing these hypotheses one by one using the data at hand. She immediately realized the data-driven decision making in this approach. I also told her that people who run their business day-in and day-out can help their data analyst with a list of hypotheses they think are plausible.
Arrange a meeting between your data analysts, business people (the people that run the business day-in, day-out), and your database admins. The goal of this meeting should be to collect as many hypotheses as you can.
I once sat in such a meeting with a marketing company that was trying to discover the marketing channel that gives them the best conversion per dollar spent on advertisement. They came up with the following hypotheses:
- Seasonality matters for some channels (TV vs online vs print)
- There is a law of diminishing returns in some channels. For example, with a TV advertisement, after a certain number of impressions per week, showing more ads for the company’s product may not yield more conversions
- When our competitor runs Google ads, we should run Facebook ads
- We are currently under-spending on marketing
- And many more…
They didn’t have to test all the above hypotheses in their first run. They picked one that they felt was the lowest hanging fruit, and built a predictive model for it. If you feel that seasonality might matter, ask your database admin to give you all marketing and sales data for the last few years on all months, feed that into your predictive model, and see if you can prove/disprove that hypothesis.
More than proving or disproving your hypothesis, you might find that the ROI on your marketing dollars are X times more in Winter than in Fall. This insight itself could help you optimize your marketing budget and save a ton of money for your company.
A small win like this can get the ball rolling for you by getting more supporters for your cause, and before you know it, your company is already on the data science bandwagon! You no longer feel left behind when someone brings up the topic of machine learning in corporate meetings or at the innovation conferences.
Summary
In summary, gather knowledge and act on it. Learn about data science, purchase an automated machine learning tool, act on that knowledge, aim for small wins, make your success your team’s success, and make it iterative.
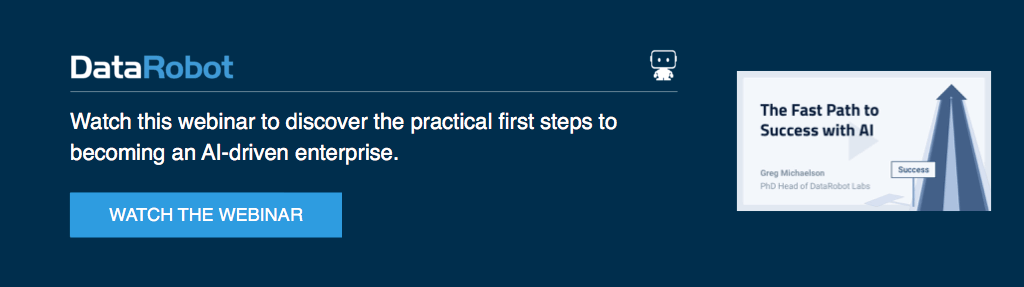
Author’s Bio:
Raju Penmatcha is a Data Scientist at DataRobot, where he helps organizations create value through the application of data science. He holds a PhD in Petroleum Engineering from Stanford University and an MBA from Wharton School of Business, where he majored in Marketing & Entrepreneurship. He has been a practicing data scientist and a technologist for more than 15 years.
In addition to working as a Data Scientist at institutions like Johnson & Johnson and HSBC, Raju’s experience includes entrepreneurship and working as a Big Data Platform Manager, TOGAF & J2EE certified Enterprise Architect, and Senior Researcher at companies such as American Express, Goldman Sachs, and Mobil Oil. Raju has published several technical papers in technology journals and presented them at international conferences.